Big data analysis as a tool for international development work
Increasingly, governments and citizens in developing countries as well as development agencies are using information technology to improve governance, enhance public service delivery, shape government-citizen relations, and reduce corruption. In particular, “big data” and “open data” on governance represent important new sources of information for anti-corruption work. However, we are just at the beginning phases of understanding how to best use these new data sources in anti-corruption work, as well as appreciating the challenges and limitations inherent in them.
Our focus in this brief is on the use of detailed administrative data that pertains to activities and individuals involved in governance, and that is suitable for use in identifying or evaluating corruption. Often, but not always, these data sources are open – meaning they can be freely used, reused, and redistributed by anyone, anytime, anywhere. And often, but not always, these data sources are big. Big data has four characteristics:
- Size or volume
- The speed or velocity at which it is generated
- The variety of forms in which the data is generated (ranging from photos to text messages to social media posts)
- The complexity of the information being created, in terms of the ability to interconnect different data sources.7df9419952a5
However, we do not restrict our scope here to only big or open data. Often, governments and other organisations keep data out of the public realm. And often, highly relevant data for anti-corruption efforts pertain to “medium” numbers of observations, such as asset disclosures of just several thousand politicians. Yet in recent years, these forms of data have also become increasingly available or “open.”
Our specific focus here is on administrative data that is sufficiently detailed. Data must be granular and broken-down to be of any use for anti-corruption purposes. We further focus on data from official sources, rather than data collected by private actors or crowd-sourced from the public, as such sources raise additional questions regarding sampling and representativeness.
Several global trends have contributed to the increasing accessibility and availability of data relevant for anti-corruption efforts. More and more governments have adopted e-government, transparency, and open data projects in recent years. New technology and global initiatives like the Open Government Partnership, the Open Contracting Partnership, and the Extractive Industries Transparency Initiative have helped to make such projects possible. These developments are pushing governments around the world – including aid-receiving governments – to shift towards a more technology-centered way of working and interacting with citizens, including the collection, generation, and publication of many new data sources. Below, we review several of these sources with applications to anti-corruption efforts.
Types of data for anti-corruption efforts
Public procurement data
As much of the world’s corruption takes place through public contracts,a0e3b96d3b4c using data to better monitor procurement and detect corruption offers tremendous promise. For example, procurement data has been used to detect networks of politicians and companies at high risk of corruption,7f68bd3a22bd measure corruption risk in contracting across European countries,c8d1679e11f8 and link campaign contributions with “quid pro quo” contract awards in Brazil and Colombia.32fd5c808769 In Paraguay, a new open procurement data platform led to public reporting on inflated education contracts, major protests, and the resignation of a minister.8c56d0f41d08 As e-procurement systems become more common around the world, and standards like the Open Contracting Partnership become more prominent, the usefulness of these data sources will only increase.
Campaign contributions data
When financial contributions are made to campaigns for corrupt purposes, they may be made implicitly or explicitly in exchange for past or future considerations. Relevant data sources – such as the Campaign Finance website for U.S. elections – thus offer the potential for linking these contributions with their “quid pro quo,” often by studying them in conjunction with data on public contracts or hiring. For example, Boas et al (2014) show that campaign finance contributors specialising in public works contracts receive a boost in contracts post-election when they donate to a winning ruling party candidate’s campaign.
Asset disclosure data
A third of countries worldwide require public data on individual financial assets. These data are useful for identifying politicians whose assets increased dramatically while in office. Researchers in India have carried out analyses of such data and have found that election winners’ assets increased by up to 5% compared to runners-up.e207afd157c2
Budget and spending data
Data on government budgets and spending can be another powerful anti-corruption tool. Comparisons between funds allocated and results on the ground often provide a means of identifying funds lost to corruption in sectors such as the health and education sectors.8b762f535c8f Broader analyses of resource allocation patterns can also identify policy biases in favour of certain groups.
Several countries, cities, and municipal governments have made their budgets and government spending information publicly available. Examples include the Government of the United States; the Oakland, California city government; and the Government of Nepal. Initiatives like the International Budget Partnership also contribute to efforts to make more – and better – data of this sort publicly available.
Bureaucratic personnel and responsiveness data
Governments are increasingly making data on the behaviour and characteristics of public servants publicly available. Such data can be particularly useful in identifying patterns of patronage hiring or political favouritism.a9311b6e187f This also includes data on bureaucratic responsiveness (how bureaucrats carry out their duties), for instance on responses to freedom of information requests in Mexico;e6237f15d1cd police event records;7e35861c858b as well as on public servant social networks.eb358d44ea74
Aid data
Development agencies themselves now make substantial data on their activities available, with help from standards like the International Aid Transparency Initiative (IATI) and projects like AidData.org. Several bilateral aid agencies that are U4 partners are also moving towards making more of their (often, big) data open, including Sweden and Denmark. Multilateral financial institutions like the World Bank are also employing more technological tools to improve data collection around corruption in aid projects as well as to enhance government accountability. For instance, the World Bank-funded Andhra Pradesh Rural Poverty Reduction Project used village-level mobile phone booths to enable people to report on funding misuse as part of its overall complaint handling strategy.
Transnational contracts and ownership data
The previous examples have all been from the public (government) sector. But the data revolution has also impacted the private sector. For instance, the Extractive Industries Transparency Initiative, an international, multi-stakeholder voluntary effort in the oil, mining, and gas sectors, requires sector operators (often private companies) to publish what they pay to governments in the form of royalties, taxes, and other payments, and for governments to publish the payments they receive from sector operators. EITI now collects and publishes a variety of country-level data on the extractive industries, and is pushing for disclosure of beneficial ownership of extractive industries companies. By 2020, all EITI countries will be required to disclose the owners of extractive industries companies who benefit from contracts in their countries. This initiative has recently received backing in law in the United Kingdom, which in 2016 required that UK-incorporated companies disclose the ultimate beneficial owners of a company.97c8e173beb9
Uses of data for anti-corruption efforts
All of these types of data offer many different types of uses. We suggest several possible approaches to using data for anti-corruption, organised by their goal and their scope, as summarised in Table 1. Across all of these, the users may be government entities, law enforcement or judicial authorities, individual members of the public, civil society groups, journalists, commercial bodies, or international organisations, or bilateral aid donors.
Some users will simply aim to identify individual instances of corruption, either to pursue legal remedy, to inform the public, or to bring political or media attention to a case. The ability to search these data sources for specific politicians, projects, or companies can be very important tools for citizens, journalists, and both governmental and non-governmental investigators. Even just identifying instances of corruption risk can support investigative efforts, or even enable automated compliance checks in contexts like public procurement.
Other users will aim to measure the prevalence of corruption more broadly, potentially involving comparisons across jurisdictions or over time. Such uses are generally more demanding in terms of technical and statistical skills, as well as knowledge of legal and procedural contexts. Nonetheless, governments, international organisations, and the media regularly seek out this sort of information. Tracking changes over time and benchmarking them against other data are particularly useful both for donors and for domestic audiences. Of course, such comparisons require both that data is available over time or across jurisdictions and that the data sources share common structure and definitions to make comparison possible and meaningful.
Finally, some users can further seek to build on these measures in order to identify the impact of a particular anti-corruption intervention. Combining comparisons over time and across entities with information on where and when particular anti-corruption efforts took place, policymakers may be able to better evaluate the effectiveness of such efforts. In the specific case of randomised controlled trials, this means using anti-corruption data to generate outcome variables for measurement and comparison across treated and untreated units for an intervention, such as trainings, audits, or community monitoring efforts.
Depending on the goal, each of these types of uses can also proceed with different scopes of data sources used. Some uses limit themselves to individual entries in single data sources, such as the assets of one specific politician, or the value of one specific contract. Other uses employ the analysis of a full dataset, such as the identification of suspicious patterns across all procurement data in a given jurisdiction, or the measurement of rates of suspected election fraud across all precincts in a given locale. Finally, the most promising uses generally involve cross-referencing across multiple sources of data. For example, patterns of politicians receiving campaign contributions from firms that later receive public works contracts may be indicative of underlying corruption or even constitute proof of illegal activity, depending on the legal context. Similarly, beneficial ownership data in global settings allows users to link it with other sources of information on financial flows that might together help better identify instances of tax evasion or transnational corruption.
Table 1: Possible approaches to using data for anti-corruption
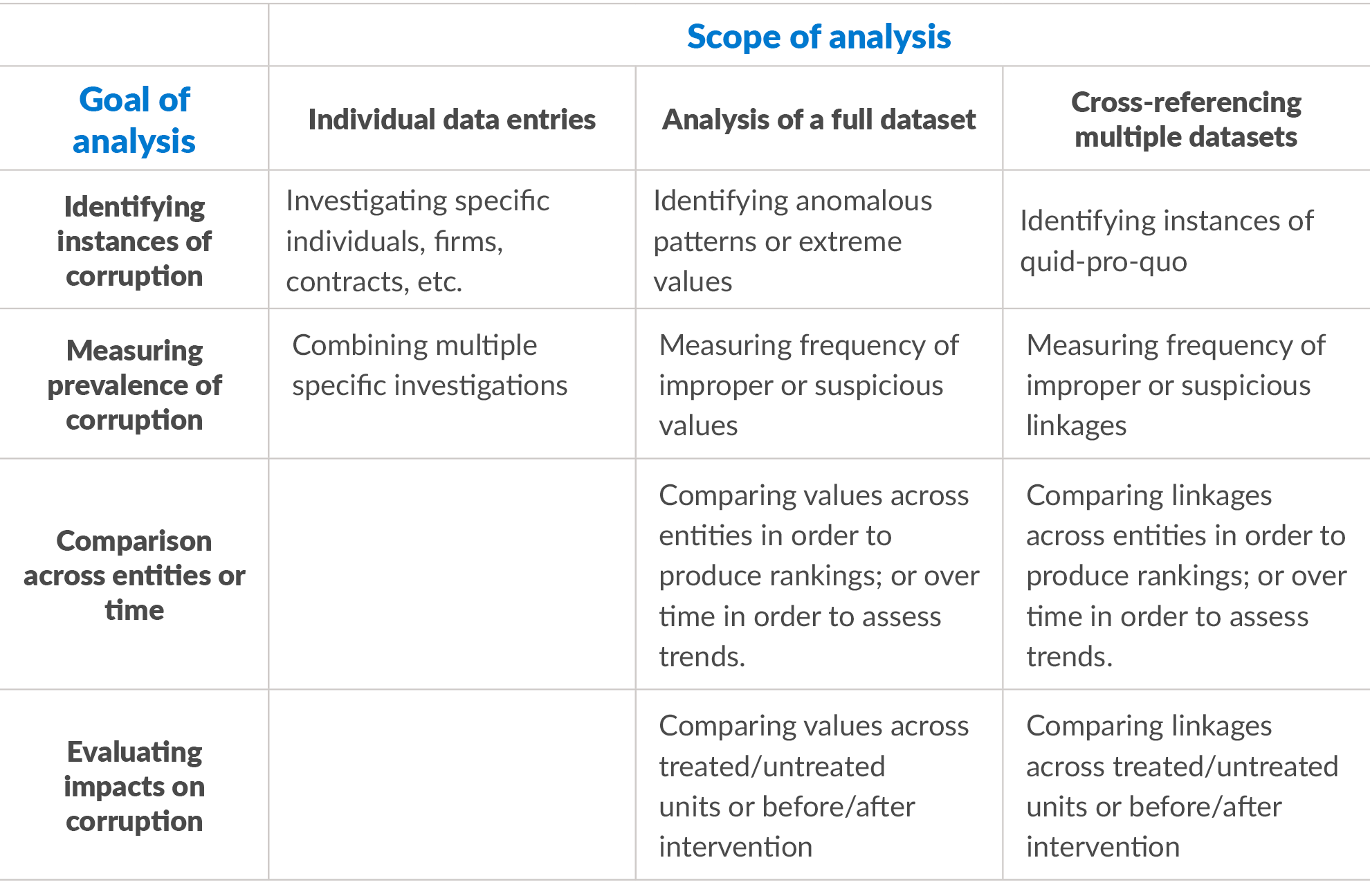
The “five Cs”: Key challenges of data for anti-corruption efforts
While open and big data sources represent a major advance in identifying and preventing corruption, these new data sources also come with challenges that that donors, advocates, and users must consider. Below, we outline “five C’s” of these challenges.
Credibility
The first challenge is the credibility of data. Information may be inaccurate or incomplete. Data from government sources may be strategically missing or misleading. Analysts must consider the organisational and political processes behind the data. Have the relevant actors fully complied with data collection or disclosure requirements? Are the relevant categories applied correctly? If there are thresholds above which disclosure is required – for example for public contracts or gifts to officials – is there reason to expect strategic behaviour to stay below the threshold?
Some of these issues may be matters of limited technical capacity or human error. But there may also be strategic decision-making behind which datasets are made public and which are not, or behind which types of information are included in, or absent from, disclosure requirements. It is essential to consider a political economy perspective439f290ad265 and question why political actors would willingly expose themselves to additional scrutiny.
Examples of such credibility problems abound. Scholars have found strategic sorting around key thresholds in cases such as procurement publicity.dec5d1bc8b1c The initial disclosures filed in the United Kingdom’s much-hailed beneficial ownership registry were found to contain large numbers of errors, including impossible numeric values and “over 500 spellings of ‘British’ ”.0667d3abe4a6 In Mexico, responses to information requests are sometimes officially recorded as “the information has been delivered electronically” when the actual response is a refusal to provide the information.77241760241a
Clarity
The second challenge is the clarity of data. Large government data sources frequently suffer from issues of format and presentation that can make it difficult, if not impossible, to actually use them for purposes of pursuing accountability. These include unclear or undefined variable names, missing identification codes that are necessary for cross-referencing with other data sources, and inconsistent spelling. Sometimes data is not sufficiently granular to be relevant for anti-corruption uses, such as when individual records are aggregated by sector or year. Moreover, information may not be provided in machine-readable format, for example when data is contained in tables in PDF documents. In other cases, data is housed in confusing or time-consuming interfaces that make it difficult to find, search, or to know what any given file actually contains. For example, in Ukraine, civil society groups noted difficulties in using a company ownership registry, including that the lack of open format meant the data was “cumbersome for analysis,” and could not “be linked with information from other databases and registries."2971b295a1df
As an illustration, consider two hypothetical countries, both with publicly available data sources on campaign contributions, public contracts, and local government spending. In the first country, these data sources all use an identical set of codes or consistently spelled names to identify politicians, firms, and localities. But in the second country, no unique codes exist to identify any of these entities, and entries in each data source use different reference codes and formats for presenting names and other identifiers, in some cases making it impossible to differentiate entities with similar names, or to match the same entity with alternate spellings. These seemingly mundane issues of clarity will actually produce high barriers – often prohibitively high – to the use of data for accountability in the second country.
Complexity
The third challenge is the complexity of data. Effective usage of data for accountability requires both a high level of technical skill and a high level of familiarity with details of the political, legal, and procedural context at hand. Users that lack one of these are likely to face substantial barriers to using open and big data sources for anti-corruption work, and they are highly likely to draw incorrect or misleading conclusions.
Uses that go beyond simply identifying individual instances of corruption will require familiarity with statistical methods and concepts, and many uses require work in statistical software environments like Stata, R, or Python. Such skills are particularly relevant where data is messy and requires substantial data cleaning or processing in order to be usable. However, technical skills alone are not on their own sufficient. Using data for anti-corruption purposes requires a high degree of legal and contextual familiarity, both in order to know what to analyse, what the results mean, and what the legal implications might actually be. Such context can be difficult for non-specialists to learn. For example, Paraguay’s procurement data portal initially found that the “only people really using the information were suppliers, economists and computer scientists." c72b6ebe527d
Often, the twin challenges of complexity mean that only large and well-resourced organisations can afford to pursue anti-corruption data analyses. In some cases, no individuals or organisations have yet attempted to use available data for anti-corruption purposes. Thus it is important to build the capacity of actual and potential users of data. There are also substantial opportunities available for creating innovative teams that combine different types of specialists.
Conclusiveness
The fourth challenge is conclusiveness: the ability to know whether a pattern is actually indicative of corruption or not. How does an analyst know what patterns actually indicate corruption, unless they already have some validated “ground truth”? Otherwise, seemingly suspicious patterns in the data may actually have perfectly mundane procedural explanations.
As noted by a previous analysis of open data for anti-corruption, “open data shows only formal aspects" of records like procurement, yet these are "usually correct in corruption schemes,” in order to divert scrutiny. Confidently identifying instances of corruption using large-scale data often requires first comparing that data with already-verified instances from some other source of “ground truth” information, such as legal cases or in-depth media investigations. Indeed, conventional machine-learning approaches are based on the identification of patterns that match an already-verified “training set” of cases. Cantu (2014), for example, first hand-coded over 1,000 vote tallies before using these to train a convolutional neural network classifier to identify likely aggregation fraud in over 50,000 other tallies. Alternately, some specific combinations of data may allow analysts to draw reasonable conclusions as to patterns that would only be present in the case of corruption – such as between campaign contributions and public contracts following elections.
Yet in some cases, the same data patterns might suggest very different conclusions in different contexts or to different analysts. Both Fazekas and Kocsis (2017) and Popa (2018) use “big data” on European public procurement with an explicit goal of understanding corruption patterns. But both face the challenge that the same discretionary procedures might indicate corruption risk in some contexts, yet the reverse in others. Thus Fazekas and Kocsis (2017) see the use of different discretionary procedures as “red flags” in different countries, and Popa (2018) finds several discretionary procedures as indicative of a stronger institutional environment that enables public officials to be entrusted with greater procedural flexibility. Without the ability to systematically compare “suspicious” indicators with confirmed cases of corruption, such inconclusiveness can be difficult to resolve.
Another example comes from Bagozzi et al’s 2016 analysis of access-to-information requests in Mexico. Although their semi-supervised learning approach can identify clusters of requests that receive suspicious patterns of denials, it still requires in-depth contextual knowledge to understand whether these are appropriate applications of legal exemptions or a misuse of discretion to avoid disclosure.
Comparability
The final challenge is comparability. Even where the other challenges are successfully overcome or ameliorated in any given context, data may still not be appropriate for comparison across governments or organisations, or over time. This is particularly relevant given that the goals of many donors and advocacy groups are to produce global measures or rankings of corruption. Varying legal frameworks, policy models, and even data structures across countries or other jurisdictions tend to hinder comparability, or even render it impossible. Details often vary as to the availability of key variables, the features that are measured, how the relevant policy domain operates, and the legal details of what constitutes corruption. These differences often mean that any country-level summary measures derived from national-level data sources cannot be directly compared.
In the case of public procurement, for example, Fazekas and Kocsis (2017) have been able to produce comparable measures of corruption risk across European countries only because of the shared legal, policy, and data structures created by a common EU standard. Nevertheless, organisations like Open Contracting are making progress in promoting common standards more broadly.
Policy and advocacy specialists have confronted this challenge in other domains as well. In the realm of access-to-information, advocates have produced comparable measures of de jure information rights in law, but never any measure of de facto compliance with those rights in practice. Despite substantial demand for such a measure, it has remained impossible to arrive at a common measurement scheme across countries, given widely varying differences in procedures, legal requirements, and monitoring regimes.
Even within countries, analysts must be wary of how differences across public authorities may confound attempts at comparison. Data from one ministry or province may appear to show more irregularities than another, in some cases not because the true degree of corruption or non-responsiveness is higher, but rather because that ministry or province faces a fundamentally different “caseload” of projects, contracts, requests, etc.
Recommendations for donors
In order for data to have a positive impact on reducing corruption in development aid, donors must continue to support efforts to generate, store, use, and publicly release data, both within their own agencies but also within the countries to which they provide support.
Developing country governments need support to digitise public records and to make their data publicly available; doing so requires technology infrastructure and training, as well as regulation of technology services and personnel. Technology hubs are emerging in many developing countries, and these could be leveraged to provide innovative solutions for data generation and storage, as well as capacity building and training of citizens, civil society groups, and government representatives.
Donors can mainstream requirements for the use and public release of data into their programmes and projects, and they can further support civil society organisations and businesses in their efforts to create applications that enable the public to generate and use data. Important attention must be paid, beyond the first-order creation of open data sources, to the more complex issues of format, verification, and usability.
Finally, donors should encourage partnerships between experts on the legal and procedural context and experts in the computational and statistical sciences in order to best leverage the potential of data for accountability. This should include pressure to look beyond the creation of new data portals and the disclosure of new types of data to the details of accessibility and verification.
- Desouza and Jacob 2017
- Paraguay’s transparency alchemists. How citizens are using open contracting to improve public spending
https://medium.com/open-contracting-stories/paraguays-transparency-alchemists-623c8e3c538f - Arvate et al 2013; Ruiz 2018
- Charron et al 2017
- Fazekas and Tóth 2014
- Lewis-Faupel et al 2016
- Fisman et al 2013; Bhavnani 2012
- Olken 2007
- Fafchamps and Labonne 2017
- Carton et al 2016
- Bagozzi et al 2016
- Bersch et al 2017; Brierly 2018; Colonnelli 2018; Pierskalla and Sacks 2018.
- See Library of Congress. Disclosure of beneficial ownership for information about other countries with beneficial ownership legal requirements.
https://www.loc.gov/law/help/beneficial-ownership/chart.php - Berliner 2014; Berliner and Erlich 2015;
- Fox and Haight 2011
- Learning the lessons from the UK’s public beneficial ownership register. OpenOwnership and Global Witness Joint Briefing. 2017.
https://www.globalwitness.org/en/campaigns/corruption-and-money-laundering/learning-lessons-uks-public-beneficial-ownership-register/ - Palguta and Pertold 2017; Fazekas and Toth 2017
- Ukraine civil society pinpoints obstacles to beneficial ownership clarity. Natural Resource Governance Institute.
https://resourcegovernance.org/blog/ukraine-civil-society-pinpoints-obstacles-beneficial-ownership-clarity - Paraguay’s transparency alchemists. How citizens are using open contracting to improve public spending
https://medium.com/open-contracting-stories/paraguays-transparency-alchemists-623c8e3c538f